Equivariant Neural Networks
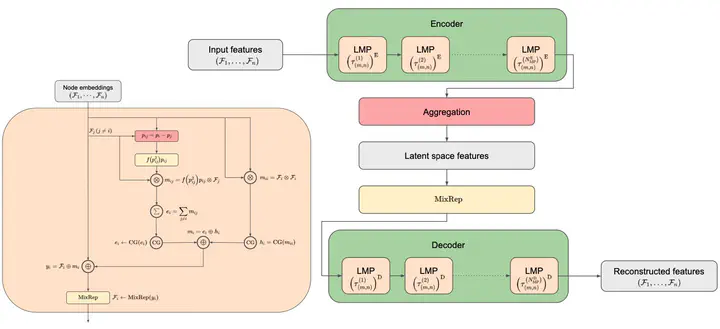
Developed a graph-based autoencoder (PGAE) for compression of and anomaly detection in Large Hadron Collider data. Wrote a review of deep learning models that are equivariant to physics-relevant group transformations for the UCSD group theory course. Led to developing a graph-based autoencoder equivariant to Lorentz group transformations as well (LGAE). LGAE outperformed CNN and GNN autoencoders for compression and anomaly detection tasks.